Whether an artificial intelligence (AI) is a genius can be measured by how well it can play board games. The new AI DeepNash announced by Google-owned DeepMind has successfully played the classic board game Stratego with human expert-level performance.
DeepNash played Stratego with humans and other AIs, beat other AIs with a win rate of over 97%, and achieved an overall win rate of 84% against human pro players, topping the year-to-date and past rankings. It is said that DeepNash finished in the top 3.
This was achieved without any key search techniques that AI has so often beaten in games like chess and Go, which is also remarkable. Stratego has long been noted as one of the challenges AI should take on because Stratego players are required to think long-term strategically, like chess and deal with inadequate information, like poker. Incomplete information means that participants are not aware of certain elements while playing the game.
DeepNash’s results are extraordinary; even the Stratego community believes it’s impossible with existing technology.
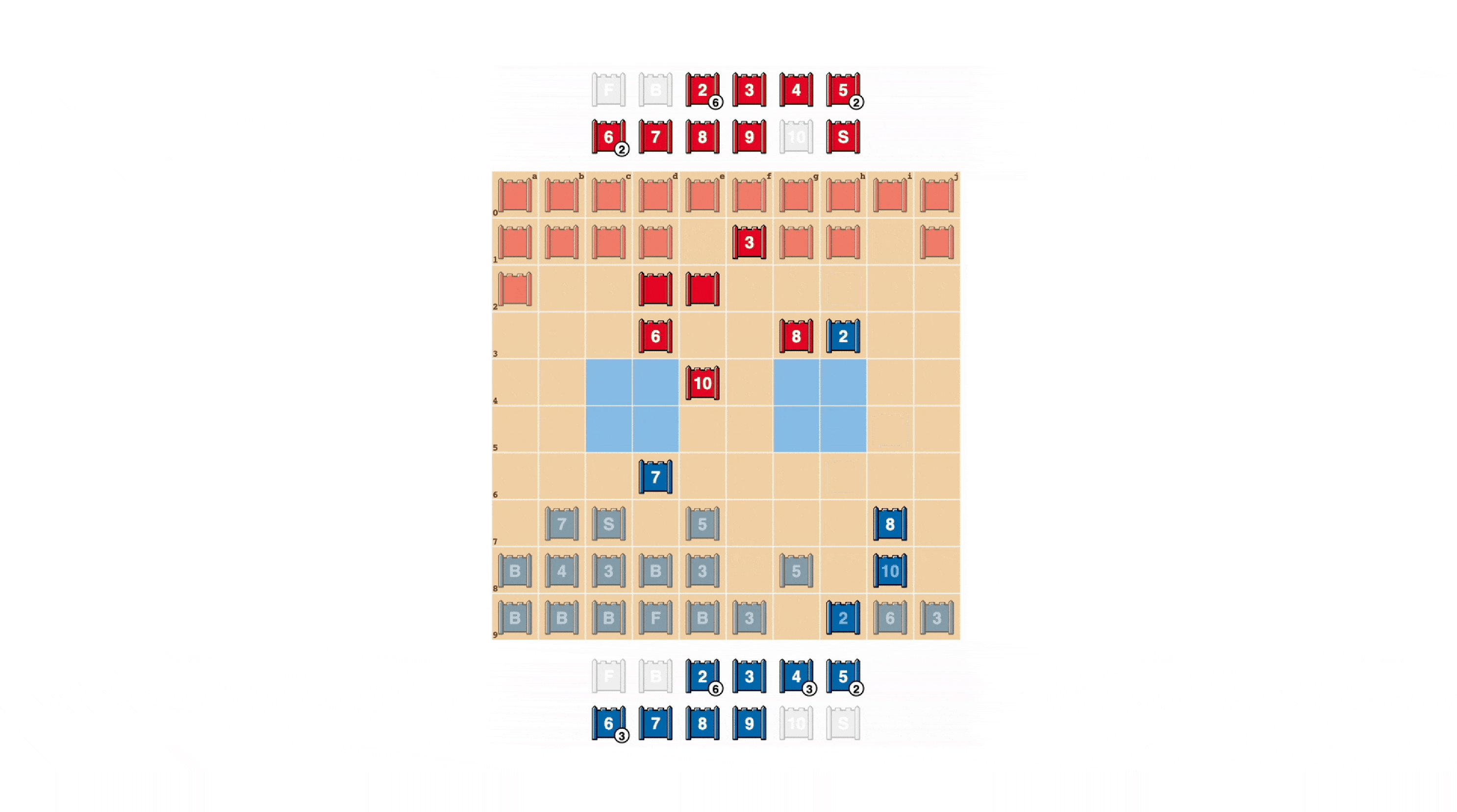
Stratego was created in 1947. It is similar to Chinese chess but has many ranks and pieces, a simple board design, no railroads, lines, or referees, and only when both sides meet are the pieces revealed and sizes determined. Both games share that victory is achieved by capturing the opponent’s flag or destroying all moveable pieces.
Stratego is an imperfect information game. Chess, checkers, shogi, go, etc., on the other hand, can be considered complete information games, as both players are fully aware of the rules of the game, the possible moves of the opponent in the current position, and so on.
The ability to plan ahead is central to the success of certain AI techniques, and imperfect information games such as Stratego allow AI to make decisions relatively slowly, deliberately, and logically in order.
DeepNash uses a model-free deep reinforcement learning method R-NaD based on game theory to learn game strategies such as bluffing from scratch without the need for exploration.
According to the research team, this study introduces a new game theory approach completely different from the latest exploratory learning methods. It relies solely on the use of game-specific heuristics.
It remains to be seen how R-NaD will develop beyond the framework of a two-player game. The method has many potential applications in imperfect information scenarios, such as crowd and traffic modelling, smart grids, auction design, and marketing problems.